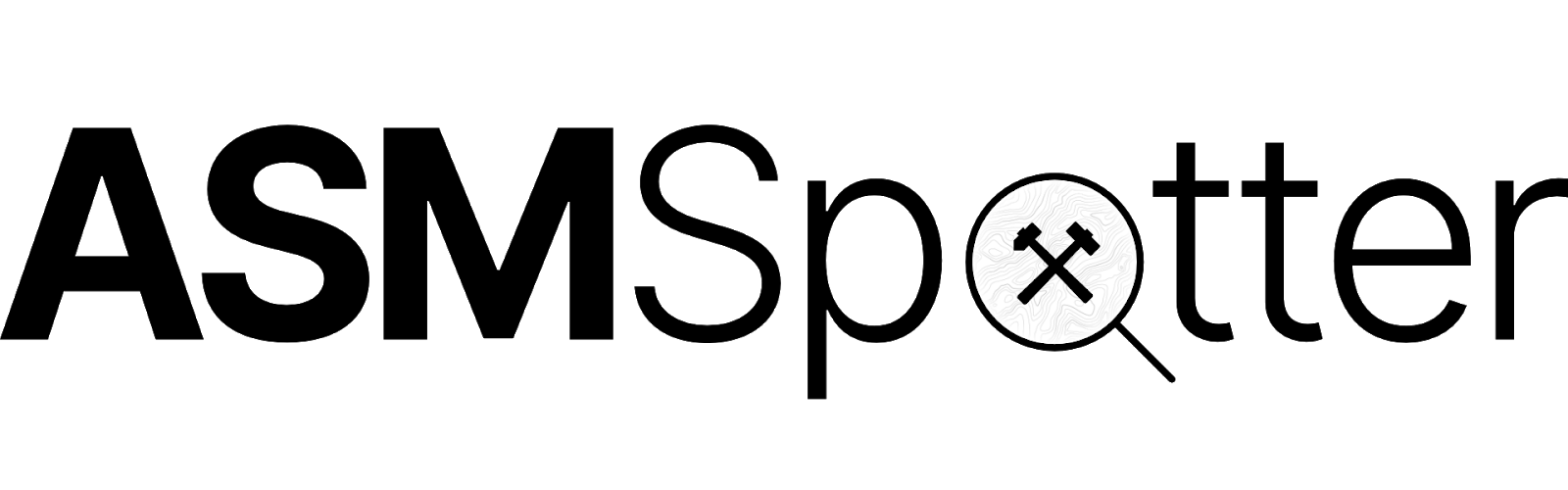
Objectives of the service
Artisanal Mining provides an opportunity for economic development for the rural population in a large number of developing countries. As artisanal mining is mainly occurring in remote areas and local authorities have a lack of resources in effective controls, illegal mining with violation of labor security standards, environmental hazards and property rights causes tremendous conflicts. ASMSpotter provides local authorities a map of where artisanal mining is occurring to prioritize controls and therefore increases the effectiveness of local initiatives to formalize the ASM sector.
Users and their needs
The targeted users are local authorities, such as mining and environmental ministries, intermediaries in the ASM sector, international and national development institutions, such as the World Bank, UN or OECD. These entities require an accurate and up-to-date picture of the situation of ASGM on the ground in order to focus their efforts for various motivations.
Local authorities:
- enforce concession rights
- monitor compliance with environmental protection and labour safety rules
Intermediaries:
- provide state-of-the-art technological solutions
- support local authorities and development institution in effective resource allocation
International development institutions:
- effective funding distribution
- tools to support local authorities in achieving program objectives
Obtaining the required information can be quite challenging and cost-intensive. So far, these identified user groups relied heavily on observations made on the ground of potential areas of interest. This could include travelling to the respective areas and/or trying to contact other entities active in the area.
As many ASM regions are located in remote areas, this poses a challenge to both material and personnel. The infrastructure is oftentimes not developed or armed conflicts make the area unsafe to travel. Even if the regions are easy to reach, it would require frequent revisits to maintain an up-to-date picture of the situation over time.
Countries where these bodies operate range from Latin America (Suriname, Peru) and Africa (Ghana) to Asia (China).
Service/ system concept
ASMSpotter leverages Deep Learning technology to localize ASM sites in the Amazon rainforest using satellite imagery. Provided with multispectral satellite images, ASMSpotter computes a semantic segmentation mask of said image. A segmentation mask is a pixel-wise classification of the image, i.e. each pixel is classified to represent an
ASGM site or not. This allows the user to not only localize ASGM sites, but also to estimate their spatial extents and shape.
At the current stage, the images need to be provided by the user manually. ASMSpotter loads the data from the user’s hard drive, computes the segmentation masks and writes the results back to the disk. The information can be used to visually represent the results.
Space Added Value
For the kickstart activity, ASMSpotter relied mainly on high-resolution multispectral images provided by the PlanetScope constellation, operated by Planet Labs. PlanetScope delivers RGB+NIR-images at a resolution of approximately 3m per pixel. PlanetScope further provides daily coverage of the entire globe.
This allows to chart ASM in the same spatial resolution as these images and with sufficient time-resolution of a few days to weeks.
Additionally, Sentinel-2 was considered as a source for multispectral satellite images. In terms of applicability, the segmentation masks computed on the basis of Sentinel-2 images resolve ASM sites similarly well compared to those using PlanetScope, although they reduce the running costs of ASMSpotter by a margin.
Current Status
ASMSpotter is a Deep Learning tool to identify and localize ASM sites in the Amazonian rainforest for northeast Suriname.
A Deep Learning model was designed, implemented and trained on a specifically compiled training dataset. The training dataset consists of
>10k high-resolution satellite images labelled by a mining expert. The Deep Learning model identifies the ASGM sites with high accuracy by computing segmentation masks of the satellite imagery.
In the future, ASMSpotter can be extended to function in other countries and with other mining techniques.
Furthermore, in this project users were identified and their needs were analyzed. To this end, a questionnaire was designed and 10 people at various institutions from the ASM sector were interviewed. This established multiple relationships and potential partnerships to develop the project further in a demonstration project.