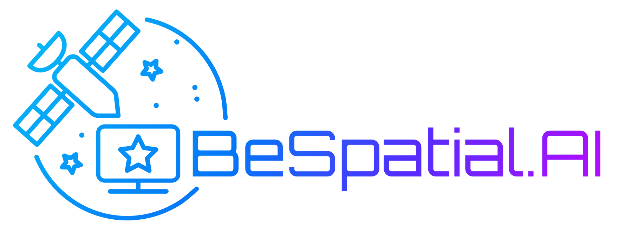
Objectives of the service
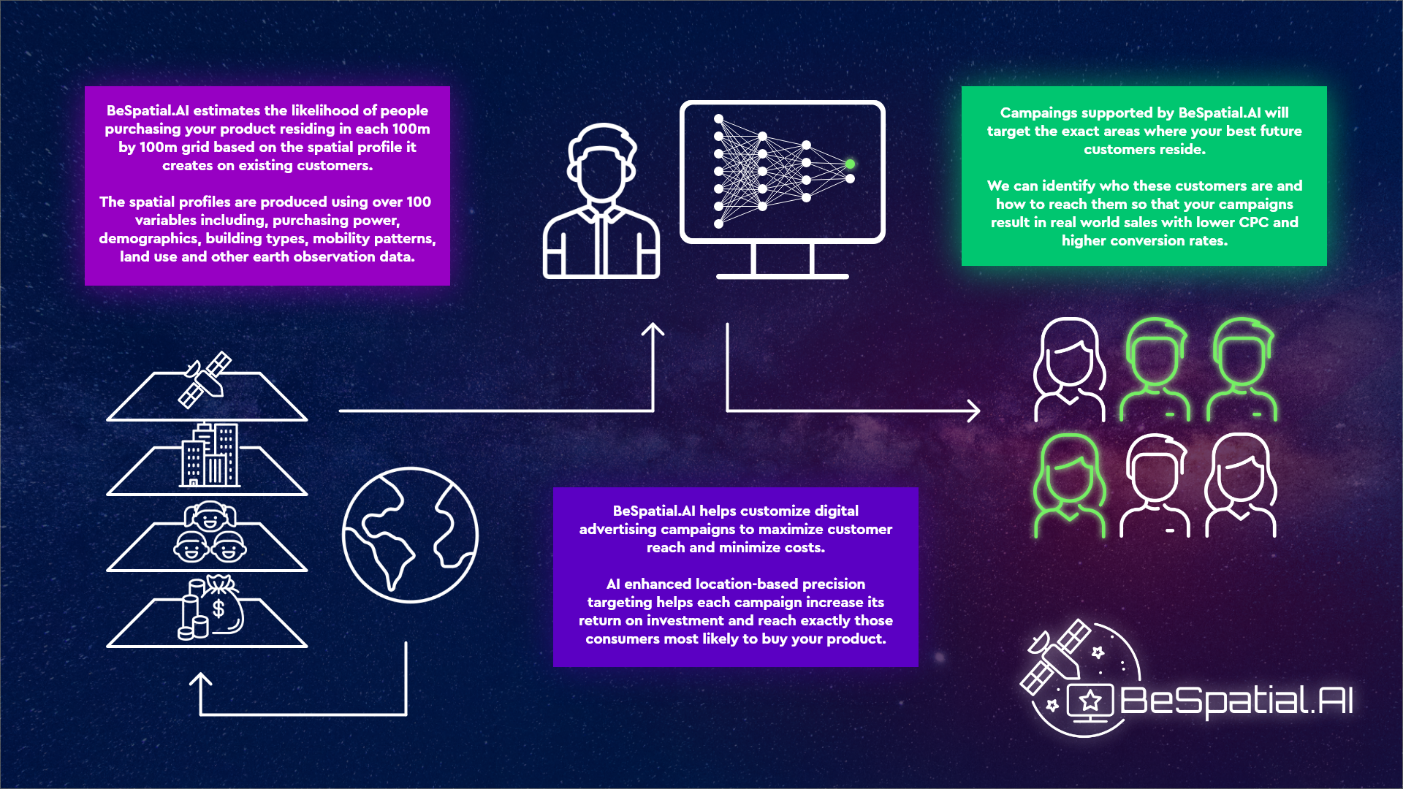
BeSpatialAI provides a solution for advertisers to leverage Earth Observation and geodemographic data assets to increase advertisement targeting accuracy by identifying the best locations to target for digital and physical ads. BeSpatial.AI is based on customer density look-a-like patterns provided by an ensemble of machine learning algorithms trained on 103 layers from Satellite and GIS data.
Good vs bad location classification model accuracy reached 91% for a given customer in FSI industry. It is not a one size fits all solution. Each client has its own machine learning model trained by machine learning model.
BeSpatial.AI brings down ads location selection time from weeks to hours.
No whitespaces overlooked, less Cost per Click, higher conversion rates, higher return on ads spent.
Users and their needs
BeSpatialAI is a B2B service for B2C organizations. We are creating value for companies in the financial services and retail sectors through making their digital advertising efforts more efficient. Based on preliminary discussion with potential customers, the direct users of our product will be the departments responsible for sales and marketing across digital channels.
Advertisers are looking for tools to better target their messaging to reach their customers reduce CAC (Cost of Acquisition) increase CTR (Click Through Rate) and ROAS (Return on Ad Spent).
The current practice in digital campaign management is to A/B test targeting, however this is a time and resource-consuming process. Often limited to creative and audience selection. Locations are rarely part of test matrixes.
BeSpatial.AI enhances targeting accuracy based on geolocational similarity AI models and customer affinity maps.
In our test campaigns we could multiply CTR, Time Spent on Site, ROAS. By finding the best locations to target based on BeSpatial.AI targeting.
Automated MLOPS process doesn’t require man months of scarce internal resources (IT, BI, DataScience, CRM) involvement.
Service/ system concept
BeSpatial.AI provides the locations to our customers where they are the most likely to reach their target audience so they can target their advertising efforts more efficiently.
The system does this by analysing – through the use of Artificial Intelligence - the locations of existing customers and finding new locations that have similar spatial characteristics. When doing the analysis and comparison the system takes into account very large numbers of variables that can be used to describe a given location. These can include sociodemographic variables, land use, mobility patterns, distances from POIs, earth observation data, housing types, etc.
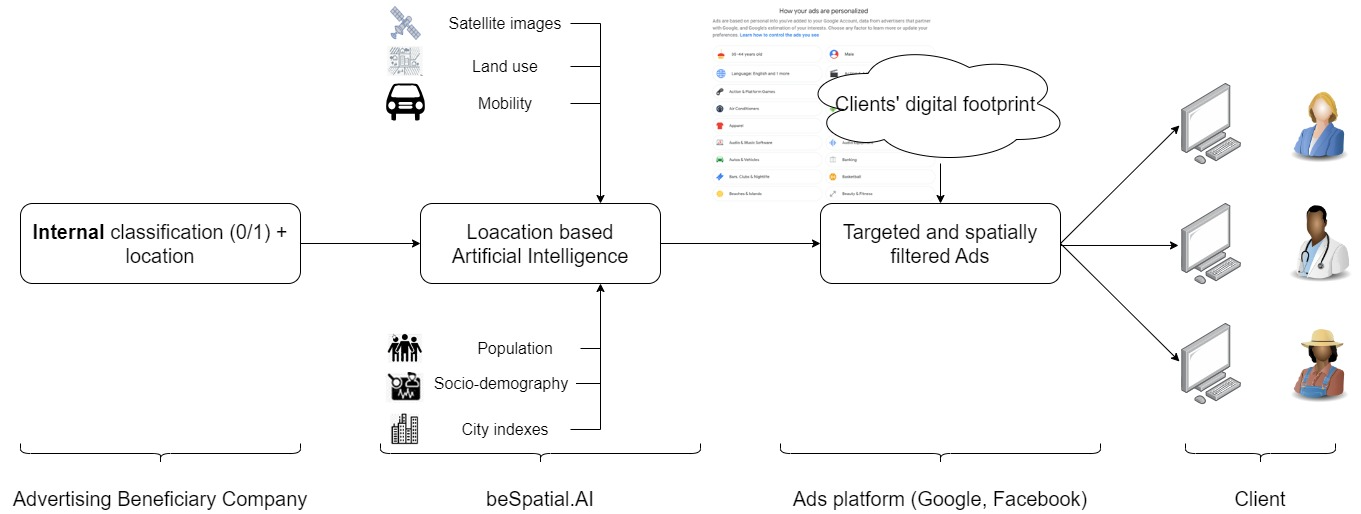
Space Added Value
In the project the following space asset-based data sources were used:
-
Sentinel-2 remote sensing data,
-
Copernicus Land Monitoring Service data sets.
This type of utilization of the space assets is a new approach, different from the “traditional” agricultural, environmental, and land use change usage of remote sensing data.
Space assets were used with the expectation that they will enhance the overall predictive power of the model and increase accuracy. In practice this has been proven correct. The space asset based variables have a significant contribution in the performance of the model.
The Sentinel-2 based indices have significant additional power in the model besides the traditional ones.
Besides this, the Sentinel-2 based indices can be used to predict population and building types in countries where these variables are unavailable.
The initial version of our model also confirmed that space assets can also be used as a substitute for certain data types increasing scalability and facilitating entry in new markets in the future.
Current Status
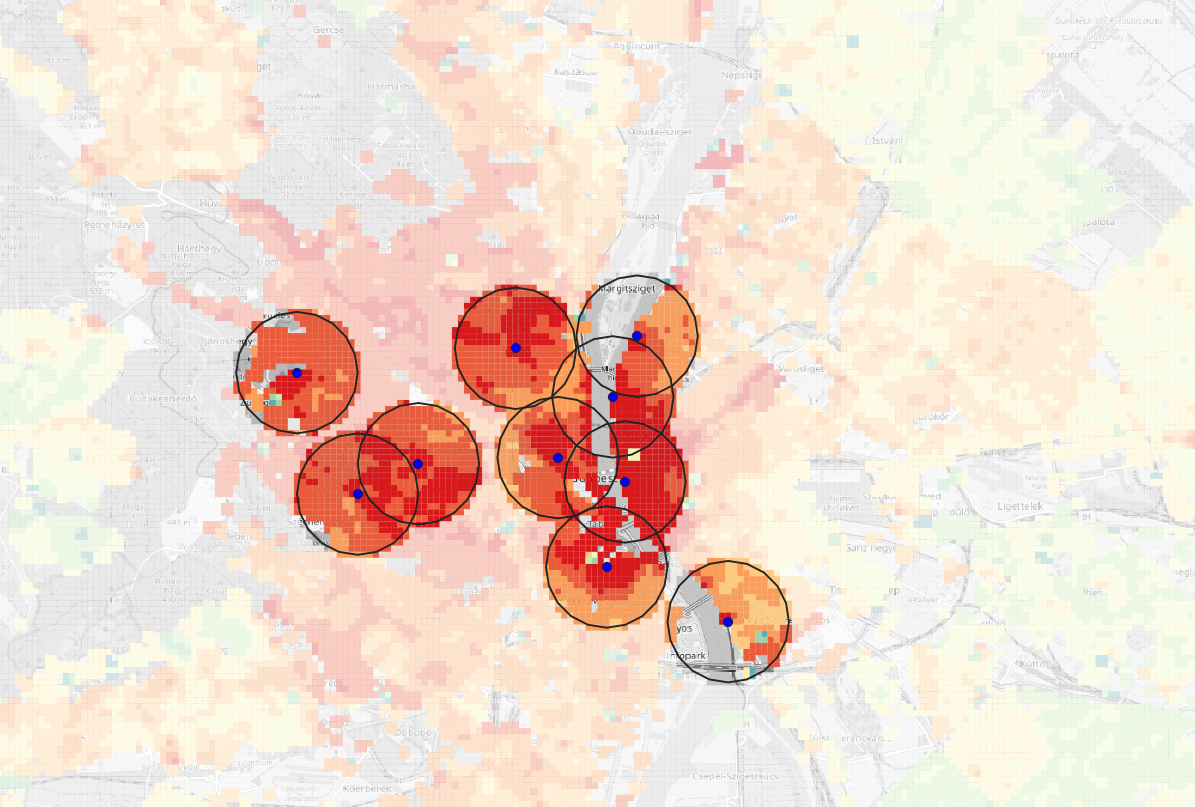
Areas of maximum product affinity per population for Google/Meta ads campaigns
We have collected potential use-cases from largest financial institutions, banks, insurance companies and retail chain operators. Objective of these (e)meetings was to identify how space assets could improve business performance focusing on sales and marketing activities.
BeSpatial.AI proof of concept codebase has been successfully developed. Even with some basic parameters hyper tuning, very close to ground truth, high accuracy heatmaps were created. While kick start project focused on market and technical core component validation. We will create a software as service for end users (advertisers) with GUI during BeSpatial.AI demonstration project.
We have successfully developed a set of proof of concept machine learning model to classify and identify good and bad locations with a stunning 91%+Accuracy distinguishing good and bad locations from customer density perspective. It required more than 4153 model training / runs testing various code versions and configuration settings. Scalability automation would require more finetuning but the technical feasibility has been proven. The algorithm used can predict potential customer density with outstanding accuracy based on Earth Observation data.
Prime Contractor(s)
Subcontractor(s)
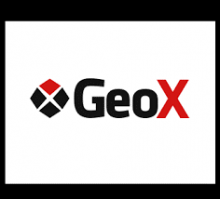