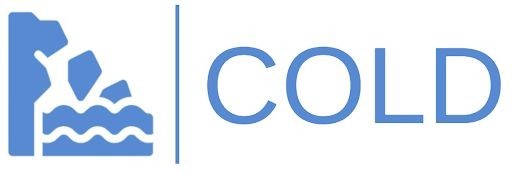
Objectives of the service
So far, the generation of near-real time Calving Front Lines (CFLs) has not been economically sustainable, because it is largely based on manual operations. No reliable automated algorithm has so far been developed, due to the complication of sea ice or compact “ice melanges” (small and large icebergs) in front of the glaciers, as well as cloud issues and darkness for optical imagery.
Thanks to its ML-based approach, COLD-ML project has implemented one of the first approaches towards the development of a fully automated, ML-based application for detecting the retreat of outlet glaciers using Earth Observations. The project is an important step forward for timely and near-real time data provision on Greenland cryosphere changes, one of the most visible global indicators of climate change. COLD-ML aims at becoming the first fully automatic application able to provide near-real time CFLs on a global scale, automatizing time-consuming manual tasks.
Leveraging petabytes of remote sensing data through employing deep learning information extraction technique, and accessing unique training datasets for optimizing ML information extraction, combined with S&T’s strong background in EO and ML, gives S&T a unique capacity to generalize and automate for offering a more cost-efficient solution for mapping of CFL.
Figure 1: The calving front line of Eqip Sermia glacier.
Users and their needs
The market areas targeted by COLD-ML are Greenland and the Arctic region. Within this area, potential users identified can be categorized in five groups:
- Commercial activities
- Fishery (and navigation)
- Tourism and leisure
- Mining, Oil & Gas
- Policy-makers and intergovernmental organisations
- National and international environmental agencies
- European Commission (EC)
- European Space Agency (ESA)
- Research and education community
- Ice sheet modellers
- Remote sensing scientists
- Climate and ocean modellers
- Thematic exploitation platforms
- Indigenous communities
Some of these users are interested in monitoring the location of CFL on a regular basis, including in receiving warnings in case of major, unforeseen events (e.g. commercial activities). Other user groups prefer receiving a CFL product to be delivered on a monthly, quarterly or yearly basis, to monitor changes in the location of the CFL to feed different types of models and forecasts (research and education institute), and/or as input to strategic environmental assessments (governments and other policy makers). Finally, the last category of identified users (Thematic exploitation platforms) are mainly interested in providing computational resources for running the future COLD-ML service and/or in including COLD-ML in their portfolio of Arctic-oriented services
Service/ system concept
The envisioned service is a fully automatic processing chain for the detection of CFLs from Sentinel-2 (S2) imagery. The only required user input is a region of interest (ROI) and timespan for each glacier. The filtering of clouded scenes and the number of scenes to be processed can be regulated via various optional settings. Processing will be triggered by S&T via a simple command line interface (CLI), specifying inputs in a basic configuration file. Processing requests are received directly from customers via mail and a predefined number of glaciers will be processed yearly. Once operational, the resulting CFL products will be hosted on one or more portals (i.e. PTEP, CVL, CCI, Cryoportal).
The core of the COLD-ML application is a convolutional neural network (CNN) model for the classification of S2 imagery into glacier and no-glacier (ocean). The CFL forms the boundary between the glacier and the ocean and can therefore be extracted from the binary classification map. The output of the application is a file containing the CFL line features with the associated date of the S2 image that was used. The Figure below gives a simplified overview of the system architecture.
Figure 2: Simplified system architecture.
Space Added Value
The COLD-ML service is based on a processing chain for the detection of CFLs from Sentinel-2 (S2) imagery. S2 satellites ensure coverage until 2024 at least. Two more S2 satellites (S2-C and S2-D) are planned to be launched in the near future, ensuring coverage further into the future. For time series extending in the past, Landsat imagery can be used.
This fully-automated, EO-based service will be an important step forward for timely and near-real time data provision on data on Greenland cryosphere changes, one of the most visible global indicators of climate change. Furthermore, AI/ML methods have a much broader potential for use across the many relevant EO missions, and digest the large amounts of data in a timely fashion.
The generation of near-real time CFLs seems not economically sustainable, because it is largely based on manual operations. No reliable automated algorithm has so far been developed, due to the complication of sea ice or compact “ice melanges” (small and large icebergs) in front of the glaciers, as well as cloud issues and darkness for optical imagery. COLD-ML aims to fill this gap, becoming the first fully automatic application able to provide near-real time CFLs on a global scale.
Current Status
COLD-ML is a service in its prototype phase and requires additional development before it can be launched on the market. During the kick-start project funded by ESA, S&T identified target markets and end-users, collecting and consolidating end-users needs and requirements. Marketing opportunities were identified and a preliminary business plan was created.
A prototype processing pipeline for the automatic extraction of CFLs from S2 imagery was developed and tested. For most glaciers the current results are already fulfilling the lower-end user requirements in terms of spatial accuracy and temporal resolution. An example of a CFL generated is given in the figure below.
A series of improvements to the core classification module have been identified:
- Class weighting.
- Inclusion of a separate class for land.
- Building a training dataset of cloudmasks in order to identify clouds.
- Integration of Sentinel-1.
S&T intend to seek funding to continue COLD-ML development.
Figure 3: Glacier/Ocean classification result (left) and the corresponding sentinel 2 image with COLD derived CFL (right).
Figure 4: COLD-ML generated CFLs for 6 major glaciers (Upernavik A,E and F are part of the same glacier) for the 2018 summer season.
Prime Contractor(s)
Subcontractor(s)
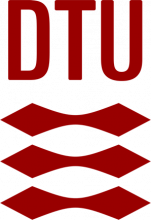
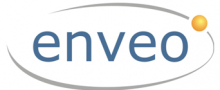