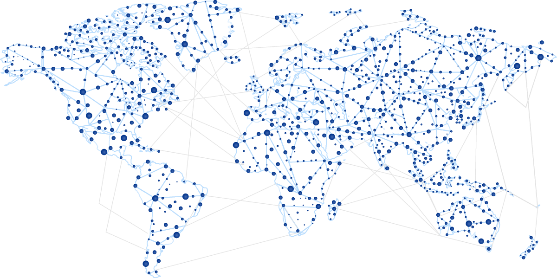
Objectives of the service
A Border Collie is the smartest dog breed. The project involves the creation of a smart algorithm applying machine learning, to look for the most efficient way to transport goods. Hence the name: Collie Artificial Intelligence.
The idea of Collie AI is to have a GPS for shipping freight. A user would input the origin and destination of cargo, and the software will provide you with the best option based on your parameters: speed, safety, impact on the environment, and costs. Collie AI would therefore be a huge step towards the realization of the Physical Internet. Having a system that is able to gather data from the myriad of sources that exist in logistics, and subsequently generate the most adequate transport plan, should be seen as a steppingstone towards the Physical Internet theory.
In the Kick start “future internet” project the Dockflow Team researched the prerequisites of such a solution and accessed its feasibility. Most of the research involved a study of the technical risks: Data availability, accuracy of the planning algorithm, and the reliability of the reinforcement learning algorithm. From a business perspective the market demand and functional requirements for Collie AI were further examined in close collaboration with users’ groups.
Users and their needs
The target customers for the Collie AI solution are freight forwarders. A freight forwarder can be described as the organization that will take care of logistics operations for a company. They are like a travel agency for transport, they plan out each route and make all arrangements (documents, bookings, insurance, etc.). The most important decision a freight forwarder must make is what routes and which transport modes to use for a certain shipment. These decisions are based on experience and habit. Freight forwarders do not have the time or resources to investigate if each transport of goods is traveling in the most efficient way. Therefore, there is the need for a software solution that helps these organizations with the decision in how to transport goods on a case-by-case basis.
Inefficiencies occurring on transports are:
- Delays
- Cargo damage (temperature malfunctioning)
- Containers getting lost
- Containers standing idle for days
Service/ system concept
The idea of Collie AI is simple, a freight forwarder inputs origin and destination, and the software will provide the most efficient route to travel. In the background an algorithm is able to gather data from numerous sources, process them and assign a weight to each data source based on it’s trustworthiness, to eventually provide a specific option on how to transport the goods. The predictions that are made should be accurate and improve each time. The reinforcement learning algorithm based on deep learning would re-analyse each prediction and re-assess to make future predictions even more accurate.
Space Added Value
Data quality in logistics is disastrous. There is a myriad of different data sources, they only show a small part of the supply chain, and often contradict each other. Collie AI would be unique by combining an unseen amount of data sources, processing their data, and being able to provide an accurate prediction. One of the most important sources for Collie AI which will allow it to offer more accurate data than other potential competitors, is the use of Satellite-AIS data. Enriching the data sources with the real-time location of a vessel, its speed, and more, creates reliable data that is unmatched by any other solution.
Current Status
The feasibility study is concluded. The conclusion of the study is to build a data cleaning algorithm with 6 specific goals
G1 | Unknown data quality issues reported by clients are reduced to zero. The algorithm warns about possible data quality issues before a client is confronted with the data. |
G2 | Reliable analyses concerning demurrage & detention, carrier performance and logistics execution KPI’s, based on structured (non-IOT) data. |
G3 | Enrichment of the analysis with AIS (space asset) and IoT sensor data. Enriching the data with real-time location data from vessels is expected to greatly increase the data quality. |
G4 | Machine-learning based analysis of the collected space data, to increase the data quality. |
G5 | Multimodal analyses: expanding the algorithm to multimodal transports. Required for Physical Internet. |
G6 | Analysis on cargo-level instead of container level. |
We will continue the development of this algorithm. This will be done in close collaboration with users’ groups: one in India, and two from Belgium. These users’ groups are freight forwarders, and clients of our current solution (a logistics visibility dashboard).