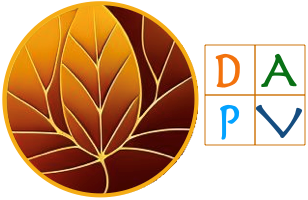
Objectives of the service
The DAPV services tries to offer a unique integrated solution for Agri-PV based using our innovative self-learning and AI-powered forecasting atmosphere system, energy system and agriculture system developed over the years powered by Satellite Earth Observation Data and actual ground data. DAPV solution would be able to forecast accurately Agri-PV site specific weather parameters, energy production, crop growth/yield, O&M and inputs for optimized Agri-PV plant design and economics.
This study is to understand the pain points of three important customer segments in Agri-PV: a) the energy companies, b) developers and EPC companies and c) large farmland owners as well as farmers. Based on the requirements the DAPV services are developed to minimize uncertainty in forecasting requirements of Agri-PV. These services will be subjected to proof-of-concept at our Agri-PV pilot users and the technical feasibility, business case and future road map will be studied and developed.
Users and their needs
Initially, the targeted customers and users are from EU countries like Germany, France, Italy, etc. The summary of important desire and requirements for most of the customers:
-
Pre-design of the system for estimation/prediction of the energy production and crop growth in the APV. There are currently no systems available for APV as a whole and DAPV services are of great interest.
-
Services should comply according to the regulations, either Crop yield first or both crop and energy production optimizations, which should be taken into consideration at the planning stage of designing the APV systems. DAPV services are well suited for such requirements and will demonstrate them in the PoC studies.
-
Predictive O&M for the APV system including losses due to soiling, cables to inverter level planning etc. for the information to farmers and the owners. The O&M service in DAPV would be quite useful for such requirements and will be demonstrated in the PoC study.
-
It would be also interesting to have the design optimized to achieve 1 – 3 points with economics of the APV plants and it is the part of the DAPV service and would be studied in the PoC.
Service/ system concept
The goal is to provide local short-to-long-term intelligent energy, crop-growth, O&M forecasting (days, months, years ahead) decision support system and services tailored for the Agri-Photovoltaics (APV) sector planning, development, economics, and their operations. Thanks to a combination of space-based earth observation (EO) data as well as PV and agriculture in-situ data, our disruptive deep-learning forecasting technology system will be able to deliver accurate energy generation, crop growth forecasts, as well as weather-based operations and maintenance insights for the APV farm owners, APV plant designer, planners, Energy companies, Agriculture companies or societies etc.
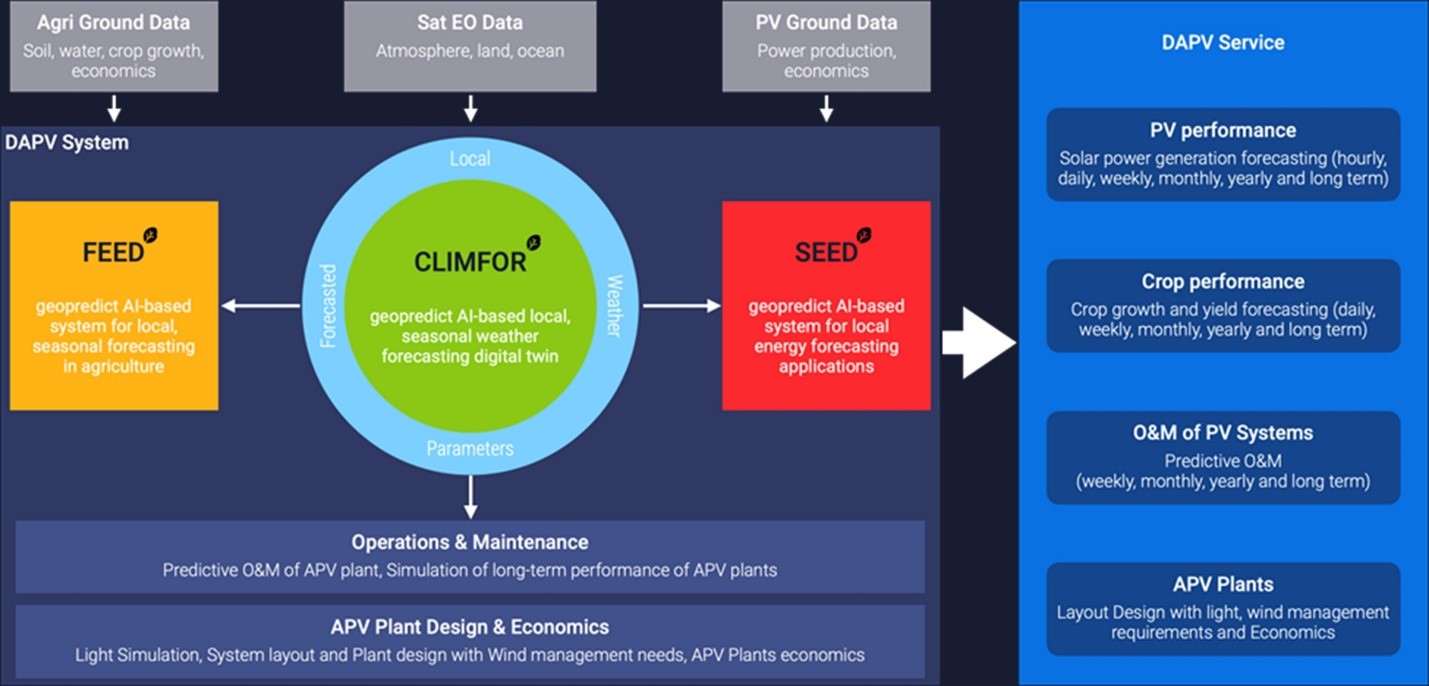
Current Status
Initial stakeholder analysis was performed for Agri-PV with identification of three customer segments: 1) Energy companies, 2) APV project developers and EPC companies, 3) Large scale farmland owners and farmers (considered User in DAPV). An initial survey and one on one consultation, about the different challenges, was performed with at least one to two customers in each segment. The corresponding user requirements were identified and mapped to the user needs and KPIs. Further, the various services required to achieve these user requirements were identified and designed.
Currently, we are performing technical feasibility and proof-of-concept of the services identified at two different pilot users in Europe at their Agri-PV sites. New updates will be done regularly on the results of this PoC and milestones.