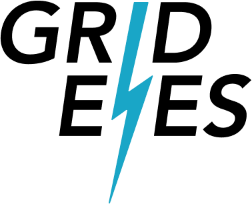
Objectives of the service
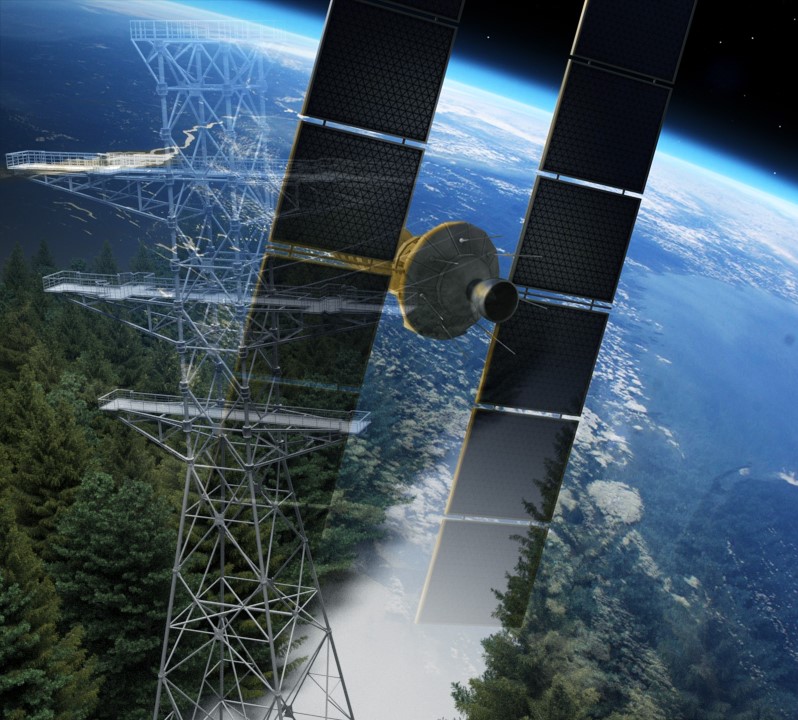
GridEyeS enhances legacy vegetation management using cutting-edge AI technology derived from Earth Observation and Weather Intelligence. GridEyeS solution is a comprehensive end-to-end process that can identify and model vegetation near power lines while estimating the risks posed by the combination of weather and vegetation.
GridEyeS addresses the challenge of processing massive and diverse datasets by employing state-of-the-art machine learning algorithms. These algorithms analyze multi-scale and multi-domain data, capturing spatiotemporal changes in vegetation.
Users and their needs
-
The GridEyeS service caters to electricity transmission and distribution system operators (DSOs and TSOs) globally, with initial users in the European and North American markets.
-
The primary users of GridEyeS are the teams within DSOs and TSOs responsible for operating and maintaining power lines, including the vegetation management, network asset management, and grid operation departments.
-
GridEyeS offers a comprehensive software-as-a-service solution covering all aspects of the service stack.
Service/ system concept
GridEyeS offers valuable information to DSOs and TSOs through a geospatial format on web and mobile applications, enabling efficient management of vegetation-related tasks and priorities. GridEyeS provides three core services.
-
Vegetation Detection: to monitor the vegetation encroachment into power lines' rights-of-way.
-
Change Detection: to detect and monitor both authorized and unauthorized construction and agricultural/forestry activities within power lines rights-of-way.
-
Emergency Management: to provide fast satellite-based insights to electric companies after extreme weather events.
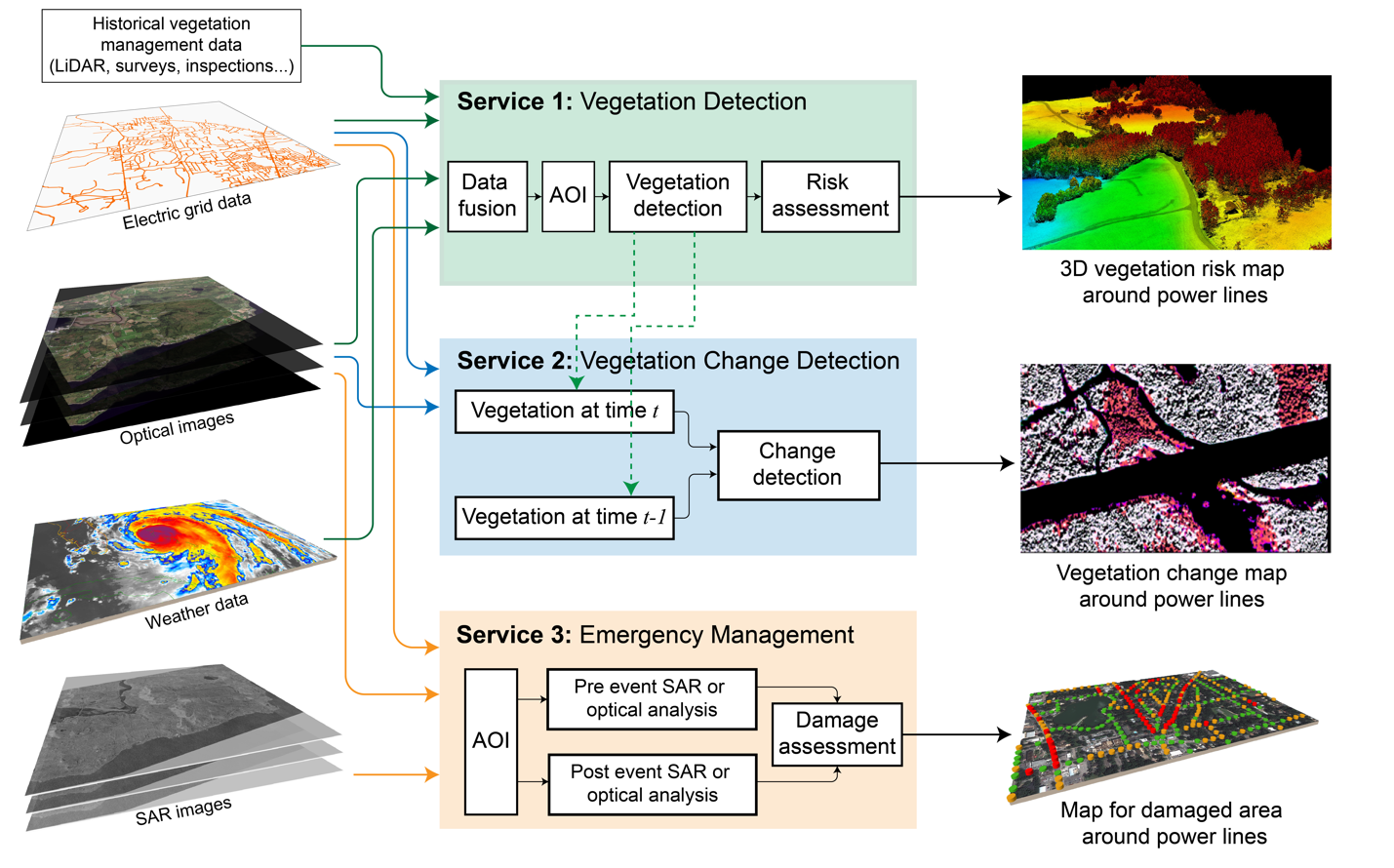
Space Added Value
-
GridEyeS benefits greatly from the valuable data provided by Copernicus including imagery from Sentinel-1 and Sentinel-2. These data sources offer extensive spatial coverage and cost-effectiveness to the project.
-
Commercial sources also play a crucial role in enhancing the capabilities of GridEyeS. High-resolution satellite imagery, both optical and Synthetic Aperture Radar (SAR), from companies like Airbus, Maxar, Planet, UP42, and ICEYE, deliver exceptional quality information.
-
By incorporating commercial high-resolution satellite images into AI models, GridEyeS can generate vegetation insights with higher spatial and temporal resolution, further enhancing its capabilities.
Current Status
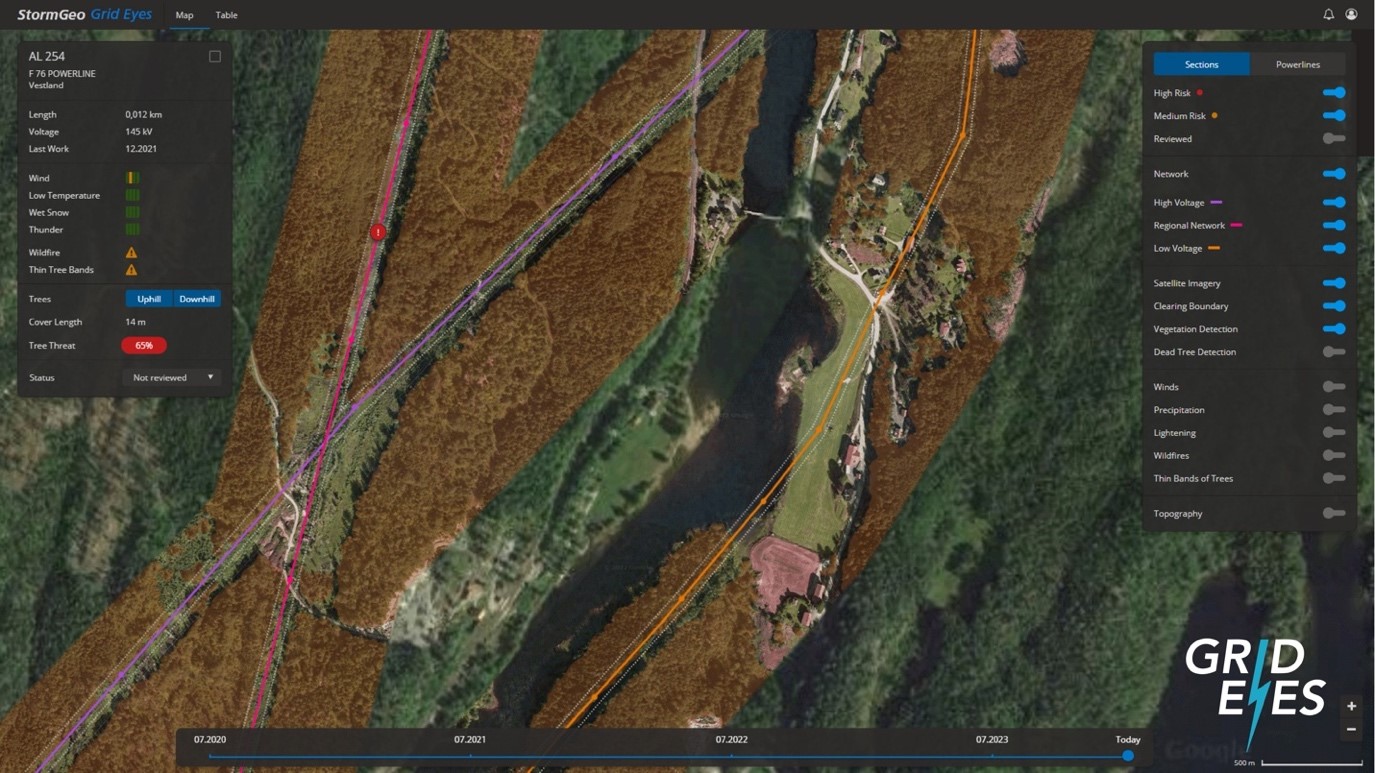
The team organized different sessions with end users in Norway and Canada to collect their needs and requirements. The BDR meeting is held. The team is moving toward CDR phase.
The project's objectives and outcomes have been disseminated to the public through Norway National TV and other media outlets, in addition to publishing the results and lessons in the professional community related to electric grids and remote sensing.