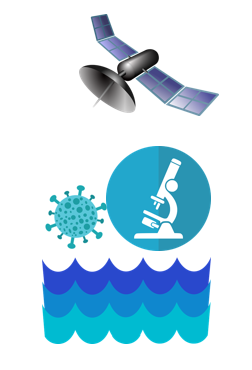
Objectives of the service
Wastewater analysis can significantly contribute to our understanding of the incidence and risk of disease in populations, caused by bacteria, viruses and other pathogens related to human sanitation. Real-time detection is somewhat of a “Holy Grail” for researchers.
Conventional methods for pathogen detection mainly rely on time-consuming sampling and in-laboratory pre-processing, careful observation and complex identification strategies. Such methods typically require assay times ranging from 24 h to up to a week. The potential presence of these pathogens requires sensitive, powerful, efficient, and ideally real-time monitoring methods for their reproducible quantification.
Advances in microscopy techniques and AI have made it possible to miniaturise microscopy solutions and train systems to automatically recognised and identify patterns, features and objects in images.
Under this activity a Proof of Concept for a novel bio-sensor based on a reduced stack microscopy technique was developed. The bio-sensor uses AI to classify, object detect, and quantify target microscopic objects.
The solution paves the way towards a real-time pathogen detection capability that will play a critical role in predicting new outbreaks of disease and also in tracking the evolution of an outbreak.
Users and their needs
The study focus is on metropolitan Pune and an urban site in South Africa as a geographic area where the possibilities of a smart sanitation and the circular sanitation economies can be demonstrated.
The concept revolves around delivering improved predictive health analytics relating to sanitation particularly in zones of the cities where adequate treatment coverage is now unavailable. While Pune is a large developed commercial centre for advanced technology employment, it also has an estimated 40% of the population living in poor housing settings in low-income (slum) areas. Basic services in these slum areas is often not available or inconsistently provided, particularly access to sanitation and proper waste management.
Service/ system concept
The overall study is to determine the feasibility of an AI-powered field deployable bio-sensor technology capable of bio-imaging water samples at microscopic scale for the automatic real-time detection of pathogens in waterways as a result of sanitation related contamination. The overall system concept is as follows:
- The development is based on a novel reduced stack microscopy technique designed to facilitate portable real time image capture on an edge-compute device.
- The microscopy images can be labelled and convolutional networks can be trained to perform classification, object detection and quantification etc.
- Optimised AI models can then be deployed to the edge-compute device for on-board real-time inference.
- Inference results can be way marked and transmitted using satellite services.
The overall system can then be deployed to waterways to provide early pathogen detection warnings that can be used instigate preventative actions to mitigate against the risk of disease.
Water borne pathogen detection - Illegal dumping of faecal sludge can result in the transmission of pathogenic materials in water courses. This can result in a significant risk to public health, particularly at low elevation levels. A floating lab deployed in the water courses can provide early warning where pathogenic material is detected.
Space Added Value
Satellite Communications are integral to our overall solution for the transmission of data.
GNSS Sensor Location and Tracking are critical from an AI perspective to understanding patterns, e.g., tracking of pathogens as a result of sewage effluent is key to understanding how sewage sludge and waste-water are moved and how they transmit harmful pathogens to local populations.
Earth Observation can be used to assess the potential for disease to spread through contaminated water and to track disease outbreaks geographically.
Current Status
Under this study, a Proof of Concept (PoC) was developed to demonstrate the overall system concept:
- A reduced stack microscopy technique was designed and a physical prototype and digital twin of the microscopy technique developed.
- Indicative image data was synthesised using the digital twin, as a proxy for real pathogen images.
- The images were programmatically labelled.
- AI models were developed and trained using the synthetic images.
- AI tools were developed include a classifier, object detector, and a density/concentration level classifier.
- AI tools were deployed to an edge compute device and successfully performed inference in real-time with a high degree of accuracy.