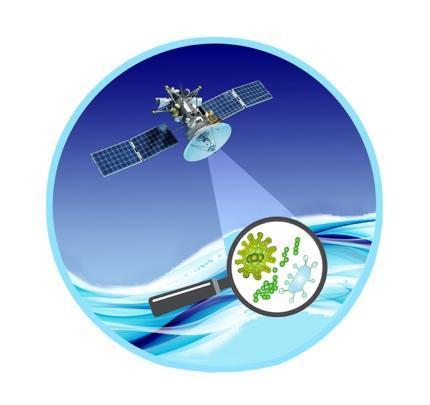
Objectives of the service
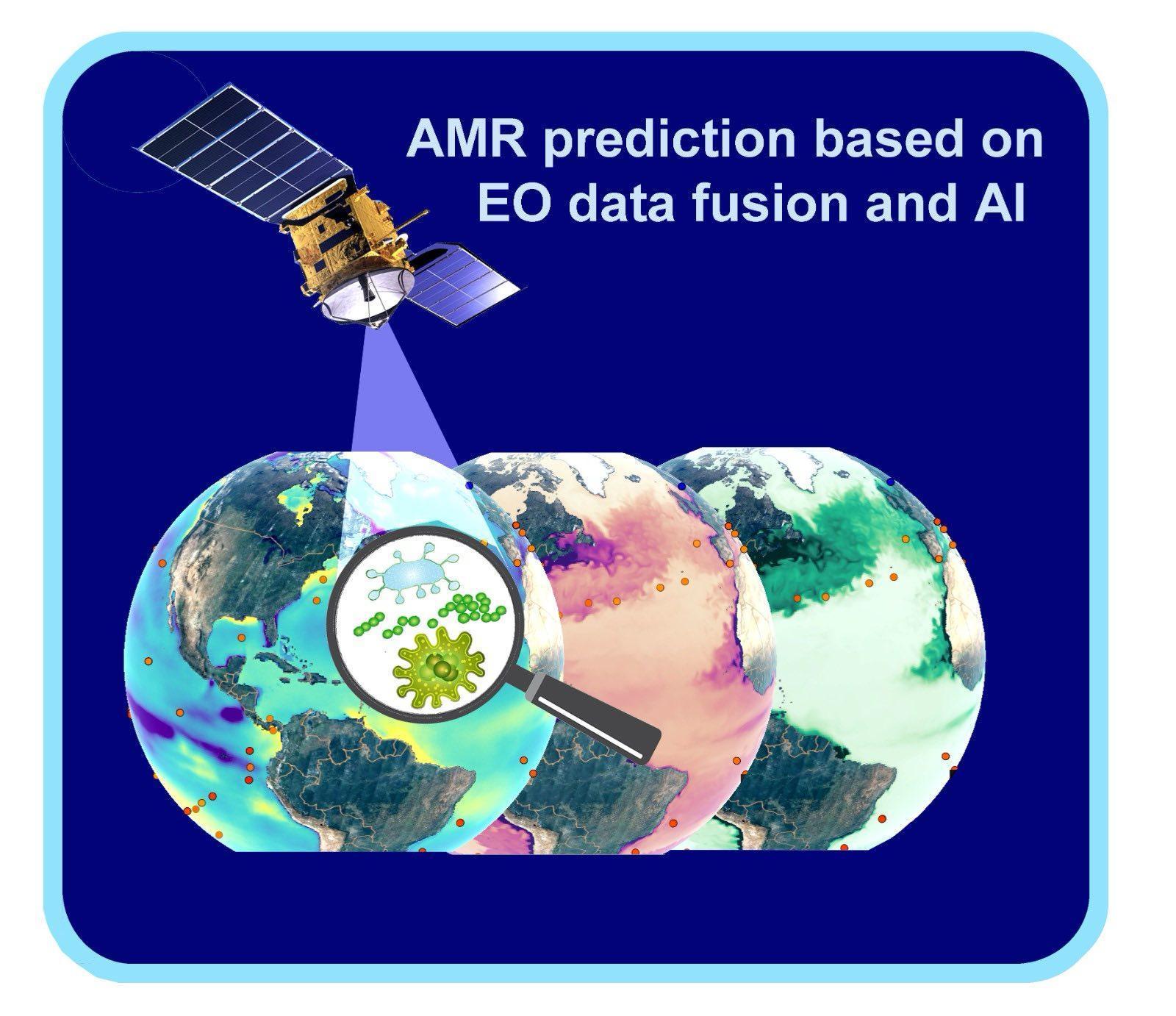
Antimicrobial resistance (AMR) is globally recognized as one of the greatest health threats of the 21st century, causing 1.27 million deaths annually. According to the World Health Organization, if no action is taken, AMR could surpass cancer as the leading cause of death by 2050. To address this, a new European Union directive mandates that municipalities with populations over 100,000 must monitor AMR levels in water by 2027.
Current AMR sampling is expensive and labor-intensive. This project aims to integrate satellite-based Earth Observation data with in-situ AMR gene abundance data to predict AMR levels in marine and coastal waters. Using biochemical and oceanographic markers from Copernicus Marine (e.g., salinity, chlorophyll-A, turbidity), the service applies machine learning models to estimate AMR abundance.
For municipalities, this can reduce the number of samples needed, pinpoint optimal sampling sites, and provide real-time alerts and pollution source differentiation. For wastewater utilities, it helps identify pollution origins and avoid misattribution. For banking & finance clients the service provides innovative & additional datasets to enhance risk model performance and access the coastal property investment.
The project built an MVP covering Gothenburg and the global ocean and validated predictive accuracy (R² = 0.98 in the Atlantic). Regional models outperformed global ones, confirming feasibility and value of location-specific AMR prediction.
Users and their needs
The Global Water Quality Digital Twin (WQDT) service primarily targets the following user communities:
-
Municipalities and Coastal Cities These users must comply with the upcoming 2027 EU directive on antimicrobial resistance (AMR) monitoring. They seek a cost-effective way to identify high-risk zones and visualize water quality trends for urban planning
-
Wastewater Treatment Plants (WWTPs): WWTPs face regulatory pressure to demonstrate AMR risk mitigation while managing limited sampling budgets. They require accurate, localized AMR risk indicators and pollution source differentiation
-
Research and Public Health Experts are our data partners
User Needs:
-
Continuous AMR monitoring from Earth Observation data
-
Identification of AMR hotspots and source attribution
-
Regulatory reporting support (e.g., EU AMR directive)
-
Reduction of unnecessary sampling costs
-
Predictive pollution alerts for public health and safety
Service/ system concept
The Water Quality Digital Twin (WQDT) is a powerful service that helps users monitor and predict antimicrobial resistance (AMR) levels and water pollution using satellite data. It combines satellite-derived water quality markers (like pH, salinity, turbidity, alkalinity and chlorophyll A, etc (more than 30 markers)) with AMR data to create AI-powered predictions, reducing the need for costly and slow manual sampling.
Users—including municipalities, wastewater treatment plants, and banks—can view near real-time dashboards, receive pollution alerts, and pinpoint the best places and times to collect AMR samples. This supports faster decision-making, regulatory compliance (e.g., EU AMR 2027 directive), and operational efficiency.
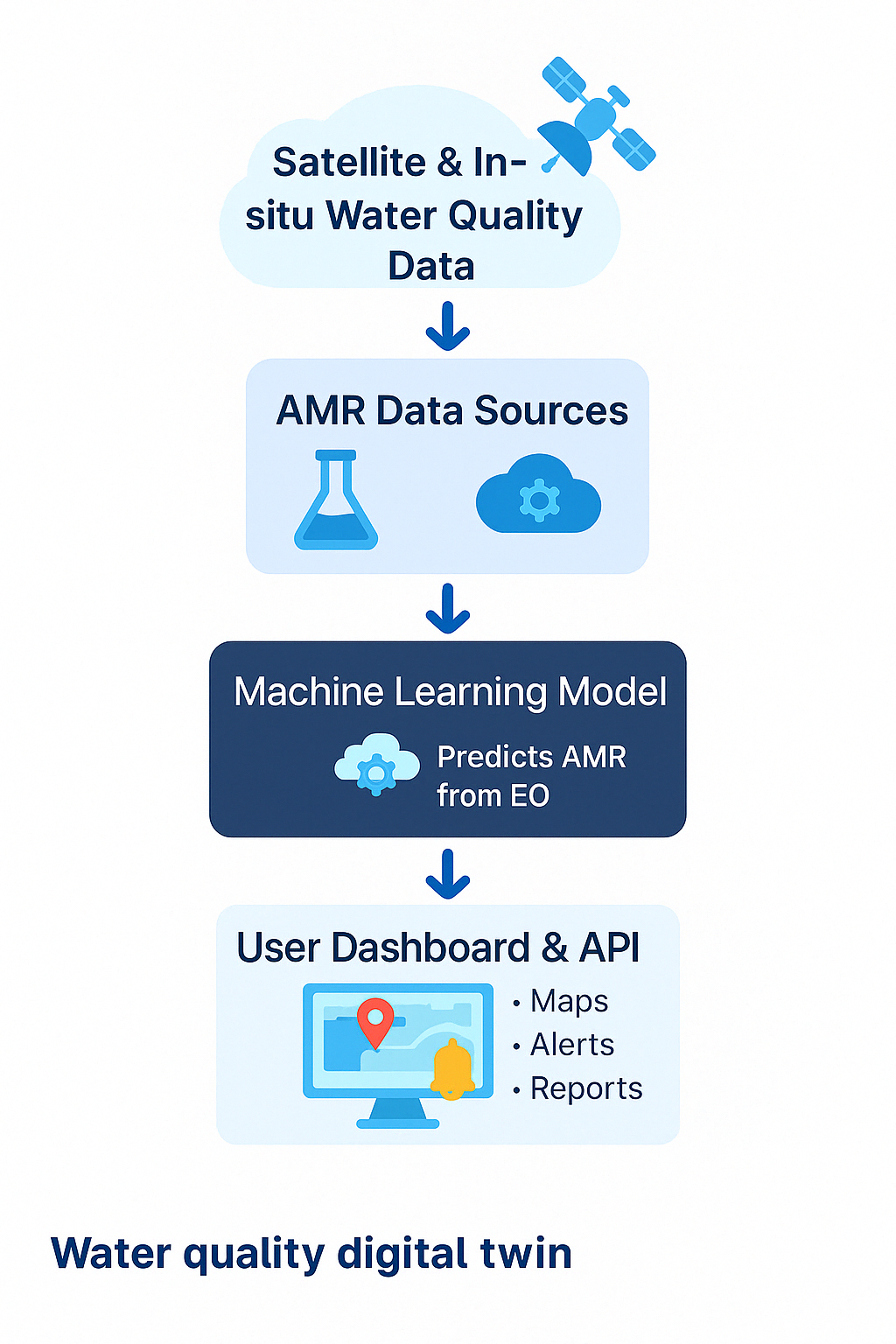
Space Added Value
Earth observation data from Copernicus Marine is essential for prediction AMR abundance levels in water. Since we use 30 water quality parameters, biochemical and oceanographic markers such as chlorophyl A concentration, water salinity, water alkalinity, turbidity water pH, etc. to predict values of AMR abundance. With our proprietary developed script by Zero Gravity Oy, we feed numeric values from 30 water quality markers from Copernicus marine to machine learning model. It predicts AMR abundance in water with machine learning models. We demonstrated that it is feasible to predict AMR abundance from EO water quality data and chose the machine learning model with the highest performance.
Current Status
Currently, we finished a feasibility study where we gathered user requirements and understood the main software features that needed to be developed for each customer segment. As well as proving technical feasibility that it is feasible to create a machine learning model that predicts AMR abundance in water from water quality markers from earth observation data. Also, we developed MVP for water quality digital twin, where user can select one out of 30 interchangeable water quality layers and observe their values by pressing on the location as well as monitor on the high-level water quality globally by using location search (https://amr.zerogravity.fi/webapp/globe). The next step for us is to secure the funding to go beyond the MVP level to higher TRLs and commercialize the technology.
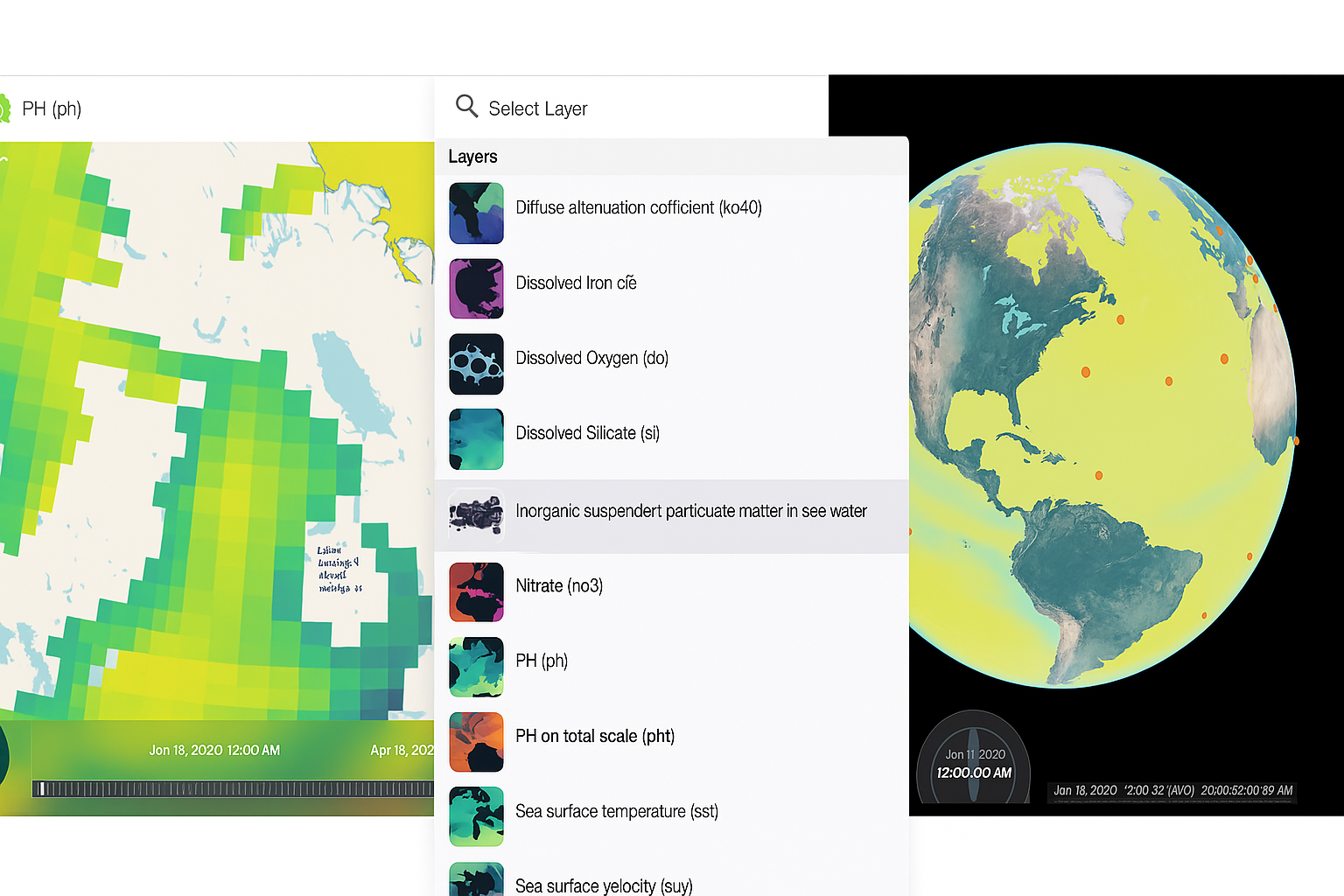
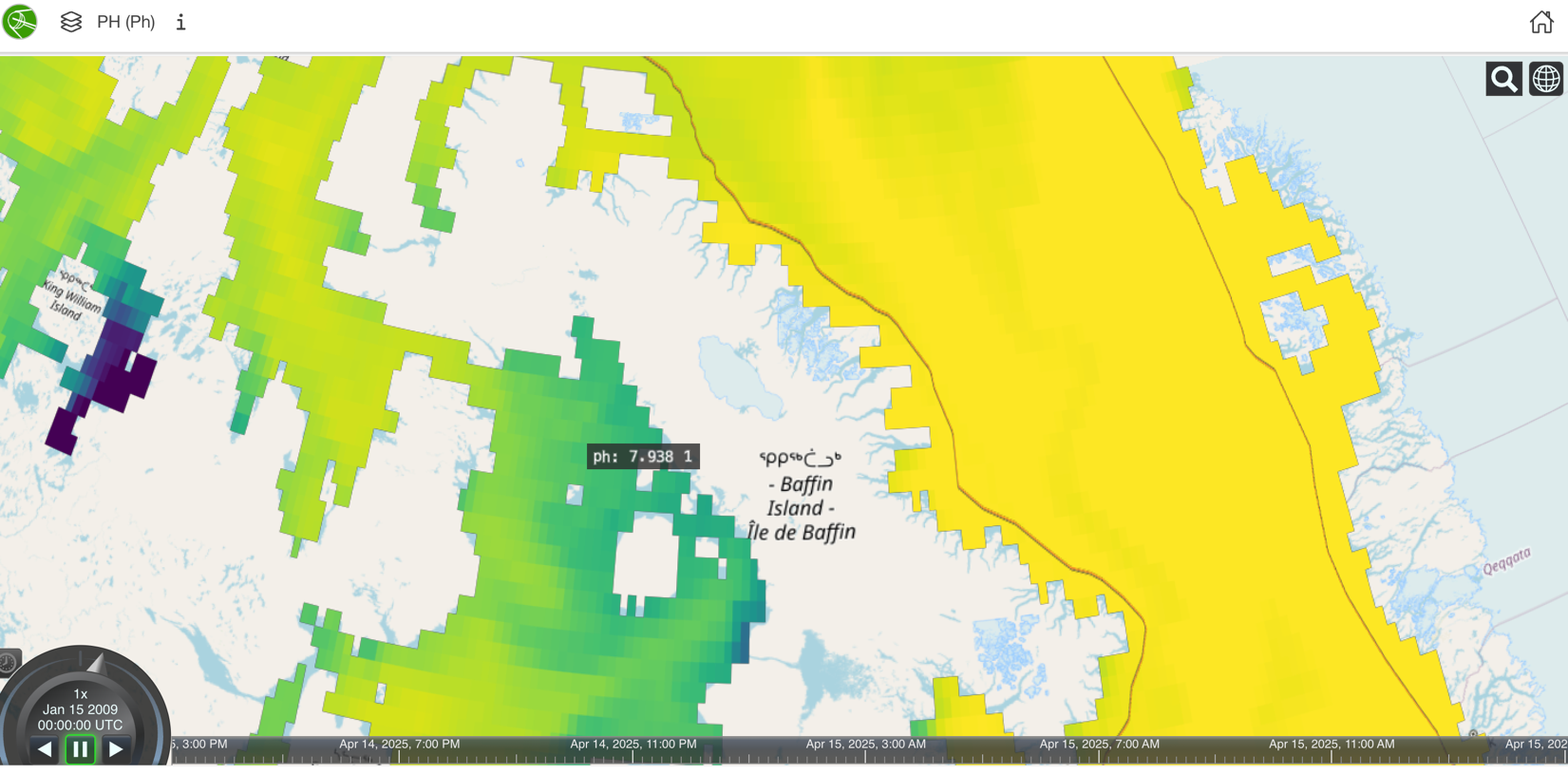
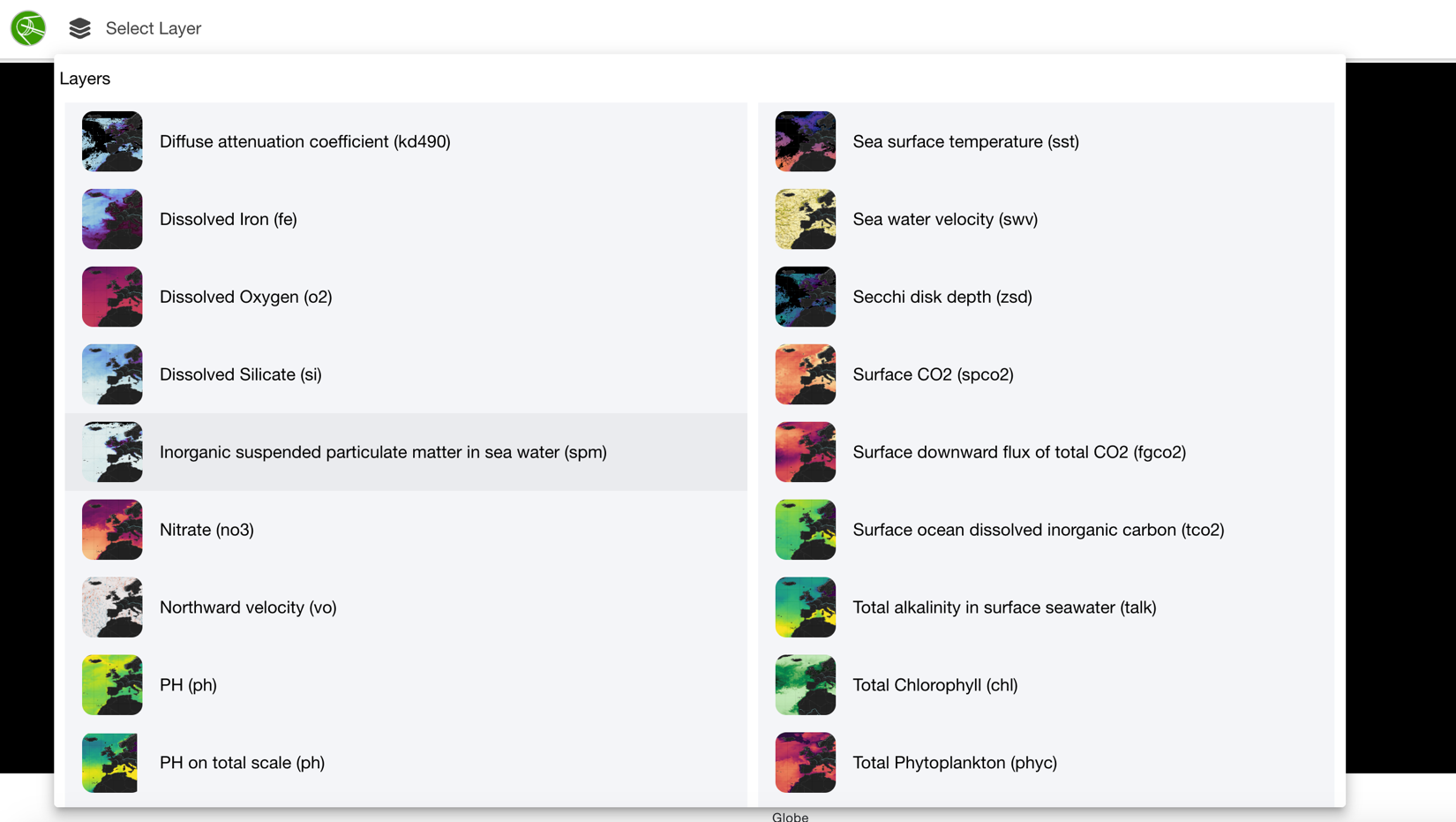